|
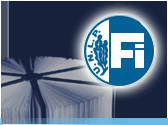 |
Biblioteca Julio Castiñeiras. Sistema de Información Integrado - Facultad de Ingeniería UNLP
Facultad de Ingeniería | 115 esq.47 | Horario: Lunes a Viernes 8 a 19 hs. E-mail: bibcentral@ing.unlp.edu.ar
|
|
|
|
|
|
|
|
|
Información bibliografica (registro INGC-EBK-000529) |
|
|
|
Título: |
Astronomy and Big Data A Data Clustering Approach to Identifying Uncertain Galaxy Morphology by Kieran Jay Edwards, Mohamed Medhat Gaber. |
Autor:
|
Edwards, Kieran Jay.
|
Otros autores: |
|
Gaber, Mohamed Medhat.
|
|
Editado por: |
Springer International Publishing :;Imprint: Springer,
|
Año de publicación: |
2014.
|
Lugar de publicación: |
Cham :
|
Descripción física: |
xii, 105 p. : il. |
ISBN: |
9783319065991
|
Colección: |
Studies in Big Data,
|
Materias: |
|
Knowledge Discovery. |
Artificial Intelligence (incl. Robotics). |
Computational intelligence. |
Engineering. |
Data mining. |
Observations, Astronomical. |
AstronomyObservations. |
|
Notas: |
Introduction -- Astronomy, Galaxies and Stars: An Overview -- Astronomical Data Mining -- Adopted Data Mining Methods -- Research Methodology -- Development of Data Mining Models -- Experimentation Results -- Conclusion and Future Work. |
Sumario: |
With the onset of massive cosmological data collection through media such as the Sloan Digital Sky Survey (SDSS), galaxy classification has been accomplished for the most part with the help of citizen science communities like Galaxy Zoo. Seeking the wisdom of the crowd for such Big Data processing has proved extremely beneficial. However, an analysis of one of the Galaxy Zoo morphological classification data sets has shown that a significant majority of all classified galaxies are labelled as â_oUncertainâ__. This book reports on how to use data mining, more specifically clustering, to identify galaxies that the public has shown some degree of uncertainty for as to whether they belong to one morphology type or another. The book shows the importance of transitions between different data mining techniques in an insightful workflow. It demonstrates that Clustering enables to identify discriminating features in the analysed data sets, adopting a novel feature selection algorithms called Incremental Feature Selection (IFS). The book shows the use of state-of-the-art classification techniques, Random Forests and Support Vector Machines to validate the acquired results. It is concluded that a vast majority of these galaxies are, in fact, of spiral morphology with a small subset potentially consisting of stars, elliptical galaxies or galaxies of other morphological variants. |
URL: |
http://dx.doi.org/10.1007/978-3-319-06599-1
|
|
|
Tapa y contenido (Amazon.com) |
|
|
El software empleado por esta biblioteca esta basado en el
Koha
Software OSS para gestion de Bibliotecas, y cumple estándares internacionales de informacion web
Número de visitantes:
|
|
|